The Significance of Bounding Boxes in Computer Vision
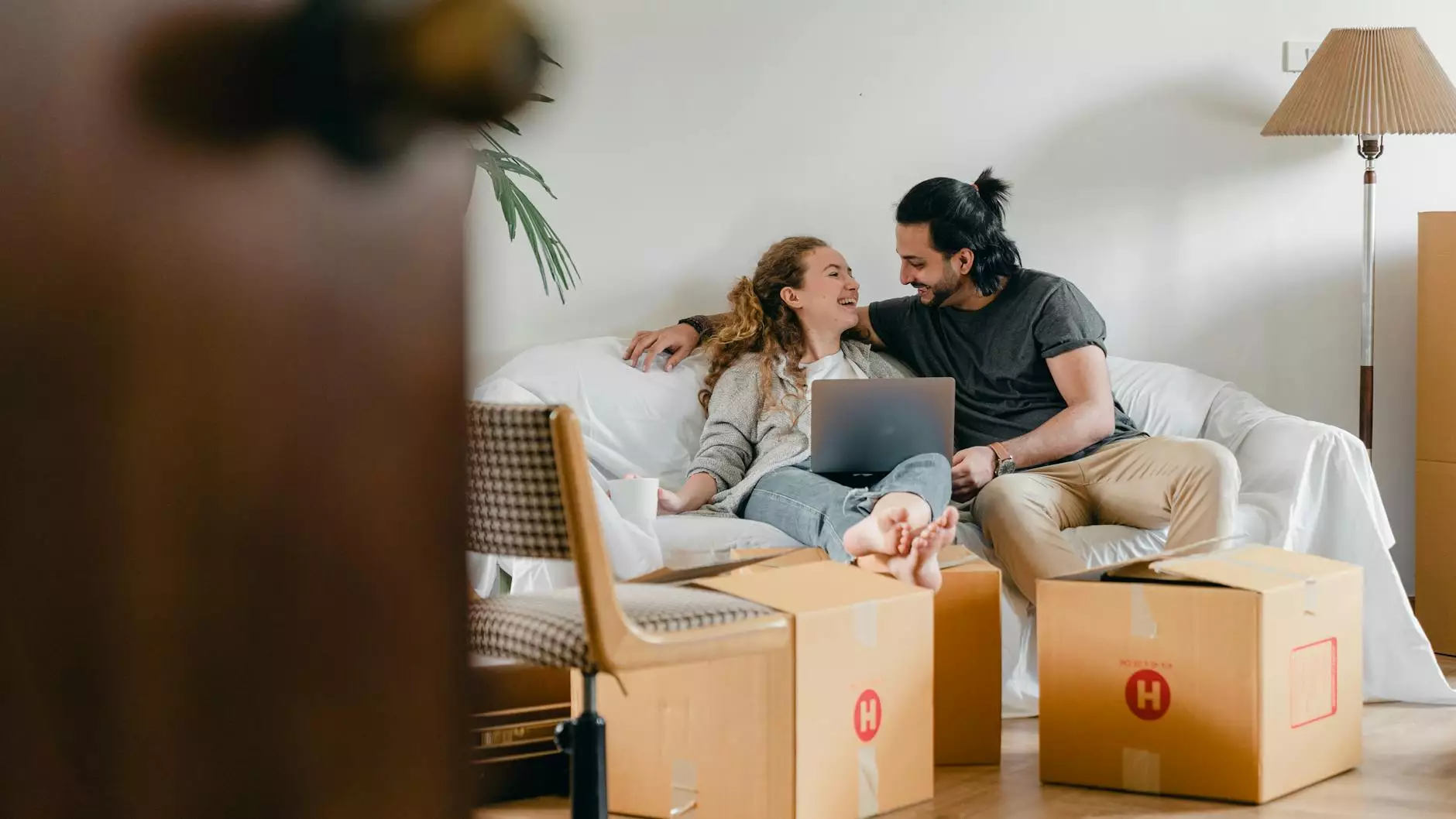
Computer vision, a rapidly evolving field within artificial intelligence, relies heavily on bounding boxes to accurately identify and locate objects within images. When it comes to tasks such as object detection, localization, and classification, the concept of bounding boxes plays a pivotal role in training models to recognize and differentiate various objects in visual data.
Understanding Bounding Boxes
Bounding boxes are rectangular shapes drawn around objects in images, defining the position and size of the object within the image. These boxes essentially create a framework that helps algorithms understand where objects are located, enabling precise identification and classification.
The Role of Bounding Boxes in Computer Vision
In the realm of computer vision, accurate object detection is crucial for a wide range of applications, including autonomous vehicles, facial recognition, and industrial automation. Bounding boxes serve as a fundamental component in the process of training machine learning models to recognize objects within images.
Data Annotation Tool for Enhanced Accuracy
Companies like Keylabs.ai provide advanced data annotation tools that leverage bounding boxes to enhance the accuracy of object detection algorithms. By precisely annotating objects within images with bounding boxes, these tools facilitate the training of highly accurate and reliable computer vision models.
Data Annotation Platform for Seamless Integration
Keylabs.ai offers a comprehensive data annotation platform that simplifies the process of annotating training data with bounding boxes. This platform streamlines the workflow for annotators, ensuring the efficient creation of annotated datasets that are essential for training robust machine learning models.
Benefits of Using Bounding Boxes
- Improved Object Localization: Bounding boxes help algorithms precisely locate objects within images, enabling more accurate identification.
- Efficient Model Training: By accurately annotating objects with bounding boxes, the model training process becomes more efficient and effective.
- Enhanced Classification Accuracy: Bounding boxes contribute to improved classification accuracy by providing clear boundaries for objects.
Overall, the incorporation of bounding boxes in computer vision workflows significantly enhances the performance and reliability of machine learning models across diverse applications.
Conclusion
In the ever-expanding landscape of computer vision technology, the importance of bounding boxes cannot be overstated. These simple yet powerful annotations serve as the cornerstone for accurate object detection, localization, and classification. Leveraging advanced data annotation tools and platforms like Keylabs.ai, businesses can harness the potential of bounding boxes to drive innovation and efficiency in their computer vision projects.